Far-sighted computer vision research
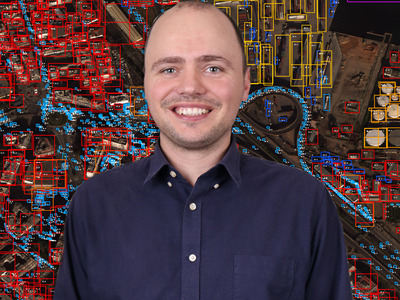
Defence computer vision specialist Dr Victor Stamatescu has developed a computer vision algorithm that has the potential to automate post-disaster assessments and accelerate search and rescue efforts, ultimately saving lives. He says it was a challenging problem because it involved scaling computer vision and machine learning algorithms, which can struggle to cope with volumes of data found in high-resolution satellite imagery.
Stamatescu teamed up with researchers at the Australian Institute of Machine Learning (AIML), which is based at the University of Adelaide, on the xView Challenge. The xView Challenge is an initiative of the US Department of Defense’s Defense Innovation Unit (DIU) and the US National Geospatial-Intelligence Agency (NGA), encouraging scientists and mathematicians to create algorithms to automatically identify objects from satellite images.
Participants were able to submit and test their algorithms against a dataset containing overhead imagery covering 1415 square kilometres of complex scenes from around the world.
Despite having never worked on satellite imagery before this, Stamatescu’s algorithm ended up ranking second out of more than 4000 submissions submitted by 100 participants from around the world. His and other top performing algorithms were 300 percent more accurate than existing government algorithms, and are now being incorporated into disaster response systems.
Challenge for large scale computer vision
The team’s algorithm uses deep learning convolutional neural network models designed for much smaller images. On the journey to their solution, team members learned important practical lessons for tackling this difficult problem. The algorithm is now being further developed for, among many other things, automated processing of full motion video in support of other R&D programs.
Stamatescu enjoys tackling difficult intelligence, surveillance and reconnaissance (ISR) problems, but enjoyed the xView Challenge because it was different to standard research where inputs and the environment can be constrained and controlled and one knows exactly what to expect.
“Our solutions stand to benefit from leveraging state of the art in computer vision and machine learning techniques,” he says. “DST is an excellent environment for this type of research, both within our team and in collaboration with universities.”
The Defense Innovation Unit (DIU) announced earlier this year that it had already begun testing winning algorithms from the xView Challenge in the wake of Hurricane Florence, assisting emergency personnel in quickly identifying flooded areas and impassable roads.