Improved vision for facial recognition
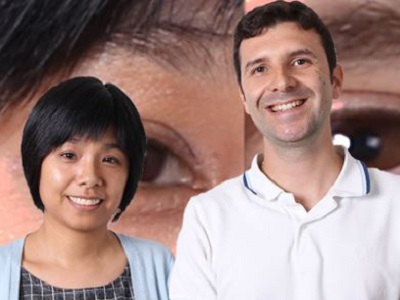
Defence scientists have enhanced a facial recognition algorithm, improving the odds of identifying someone in adverse environments such as across a distant carpark and in dark alleys.
Sau Yee Yiu and Dmitri Kamenetsky are both members of DST’s biometrics research team. “Biometrics is all about recognising people,” Kamenetsky explains. “We are asking ‘Is that the same person as this one in our dataset?’ While iris and fingerprint biometric data are the most accurate, a comparison of facial characteristics is the most common technique used because it’s reasonably accurate and CCTV footage is commonplace.”
The aim of this research was to gauge if face recognition algorithms could be used in adverse environments, for example at long distances up to 250 metres, and in really dark environments like in an alley on a moonless night.
Enhancements were made to an in-house facial recognition algorithm and then trials were conducted with long lenses across fields on bright sunny days, and in a dark tunnel facility where the scientists say they could barely see their hands when the lights were turned off.
The result? Yes, face recognition does work in these environments with the new algorithms.
Literature review directed energy
Yiu says a literature review in the early stages helped direct their energy. “I then came up with a model of how heat propagates through the atmosphere, and this turns out to be similar to the way noise from atmospheric turbulence distorts images over long distances. The atmosphere moves and shifts around and your image gets sheared and blurry. Applying my heat dispersal model gets rid of that turbulence and brings it back closer to a focused, sharp image.”
The low-light enhancement uses various filter passes to remove graininess from images.
The algorithm can be tweaked interactively, using an interface that allows several parameters to be controlled by sliders. This controls the deconvolutions applied to the images. As the user moves the sliders the output will be updated in real-time, allowing a tailoring of the algorithm to get the best results for a particular environment.
The team presented its results at the 2018 Digital Image Computing: Techniques and Applications (DICTA) conference. In the paper the colleagues demonstrate the improvements in recognition and face matching delivered by the enhanced algorithm. A further algorithm was used to calculate a metric for the overall quality of facial images. This revealed that images processed with the modified algorithm all had superior quality to the originals, concurring with visual checks.
“We’re very happy with the results, which will be of benefit to stand-off surveillance systems,” says Kamenetsky. “We’ve released a description of the algorithm, allowing other researchers to implement it and make further improvements. Interestingly, most of the research presented at DICTA was using deep learning in some way, ours is just a relatively simple yet effective mathematical approach.”
“Image Enhancement for Face Recognition in Adverse Environments”, Dmitri Kamenetsky, Sau Yee Yiu, Martyn Hole, DICTA 2018.